Tour de France Pool 2019
As I have since 2007, I am scorekeeping a Tour de France fantasy team pool. Please click here to see how poorly my "Team Florky" is doing as the tour progresses.
more ...Backyard Flowers
This spring we scattered a couple bags of wildflowers all over a part of our yard we knew we couldn't/weren't going to actively garden this year. In previous years, that part of our yard has been mostly weeds and some native grasses. We think that it looks better covered in wildflowers, and so do our local pollinators.
more ...My New MTB
Earlier this year I bought a 2018 Santa Cruz Tallboy C. This is my first full squish mountain bike, and it is awesome. The thing just floats over rocks and is much more forgiving than my old hard tail. It has 29" wheels, a 12 speed cogset, disc brakes, and a dropper post, all of which make the bike completely different from what I'm used to. Comparing times on Strava, I am faster on descents and any reasonably bumpy sections with this bike, which isn't exactly the point of getting a bike like this, but it is nice. There are trails I had stopped riding with my old hard tail because they were not fun that this bike makes fun. And that's the point.
more ...Fast Home Internet
Out of nowhere, earlier this year Centurylink started installing 1 gig symmetric fiber internet infrastructure in our neighborhood. By out of nowhere, I mean that previously they were offering 20 MB DSL service as the best option here, which is horribly uncompetitive with Comcast cable internet that we used to have. But with the 1 gig fiber, Centurylink has a product that I couldn't pass up.
And here is the wonderful result. I've never run a test where both the upload and download are close to 1 gig, but I have seen both get that high separately.
more ...Tour de France 2018 Pool
As ever, please check this link to see how poorly my Tour de France pool team ("Team Florky") is doing. Maybe one of these days I'll actually try to put a team together that performs well.
But probably not.
more ...The Wall is Done
Almost two years after the first digging started, and 10.5 months after serious and concerted work really began, our backyard wall is done!
Today I placed the last block of the wall. Hundreds and hundreds of blocks, tons and tons of rocks and dirt moved. All done!
Below a whole slew of photos covering beginning and end!
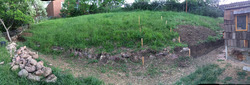
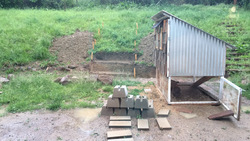
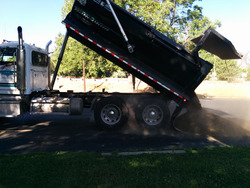
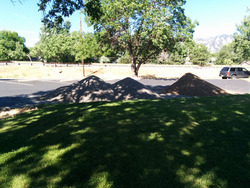
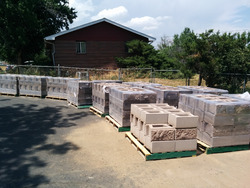
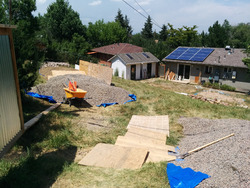
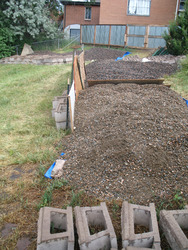
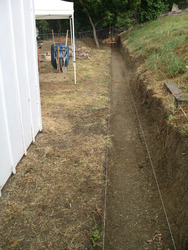
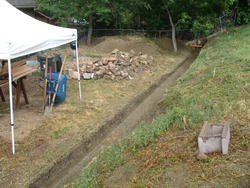
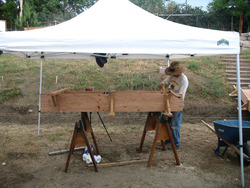
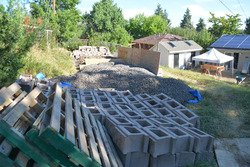
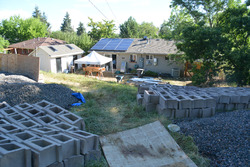
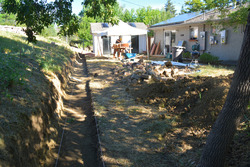
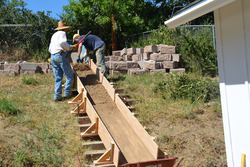
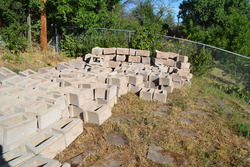
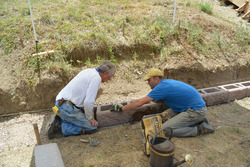
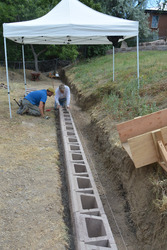
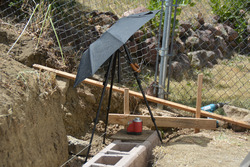
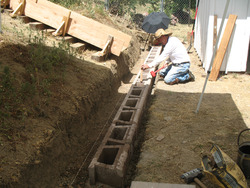
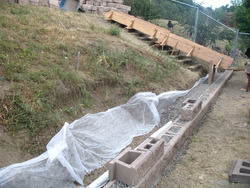
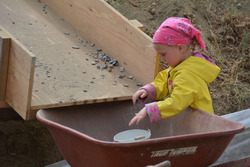
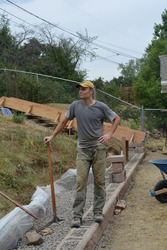
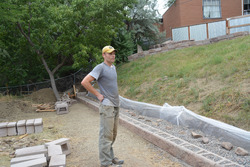
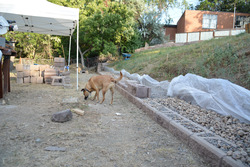
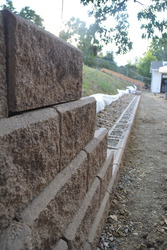
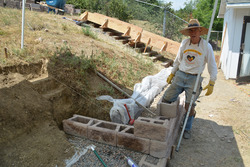
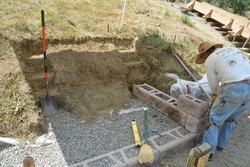
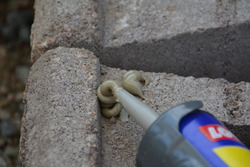
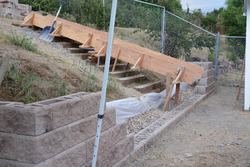
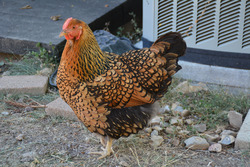
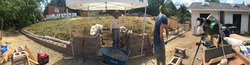
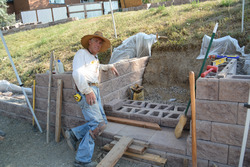
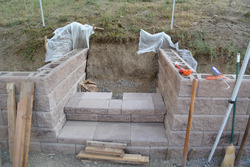
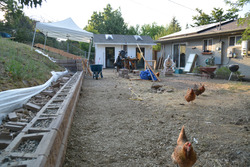
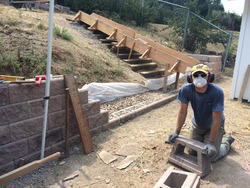
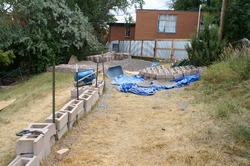
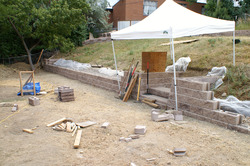
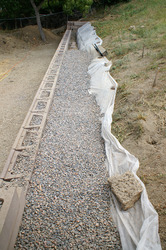
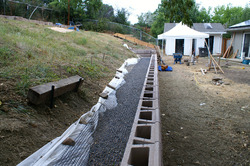
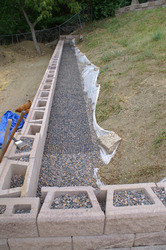
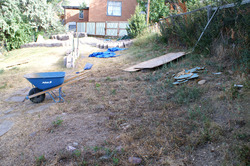
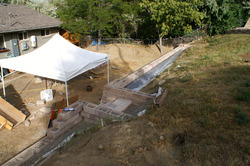
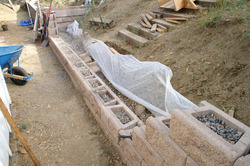
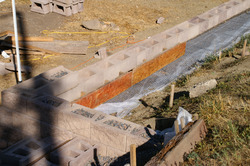
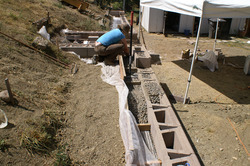
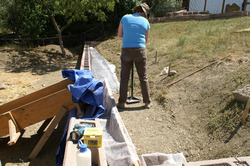
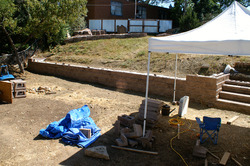
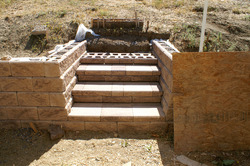
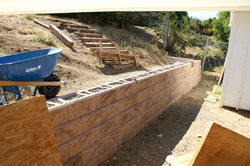
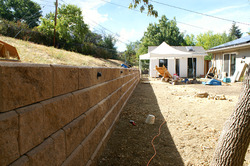
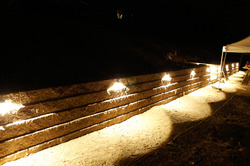
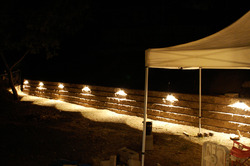
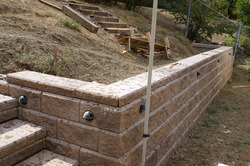
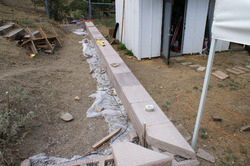
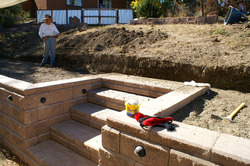
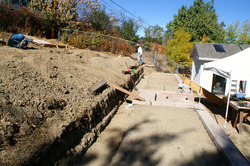
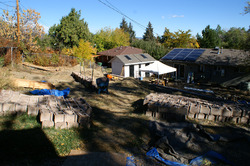
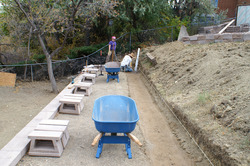
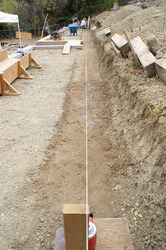
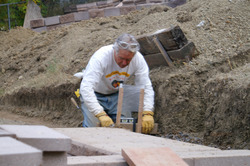
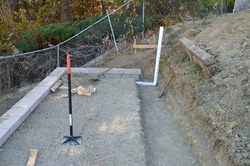
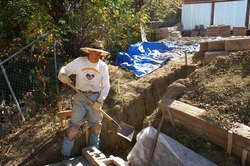
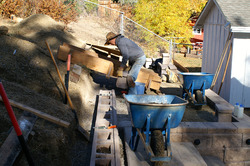
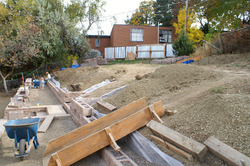
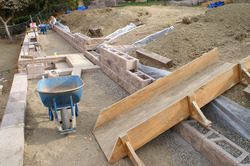
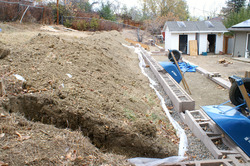
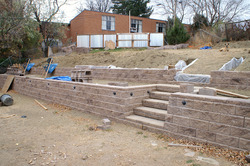
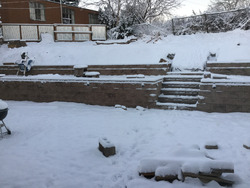
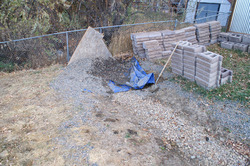
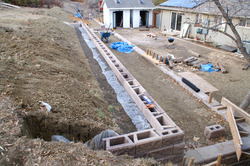
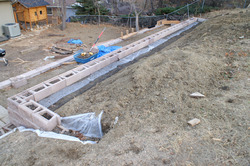
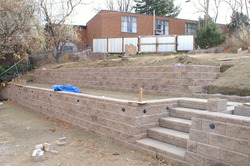
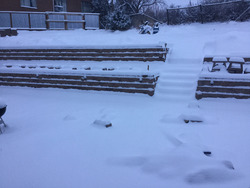
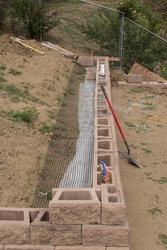
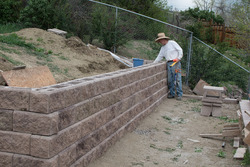
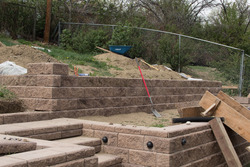
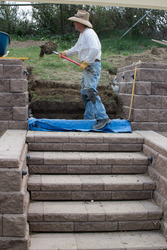
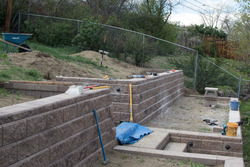
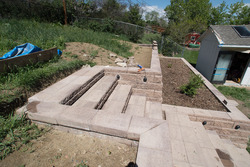
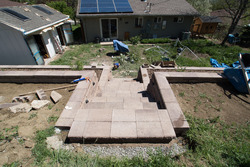
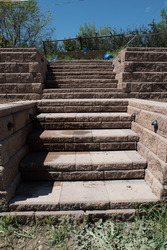
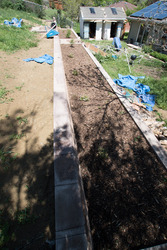
Zoo March 2017
We have a membership, so we go often, and we wanted to see the [newborn Giraffe](http://www.denverpost.com/2017/03/01/denver-zoo-baby-giraffe-dobby-born/). There is also a new Tiger exhibit, which is quite nice.
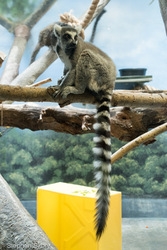
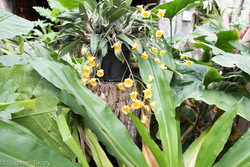
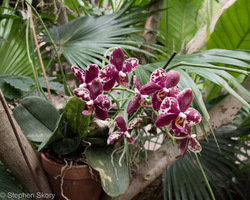
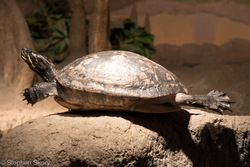
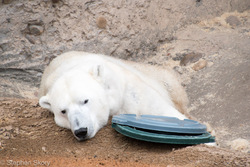
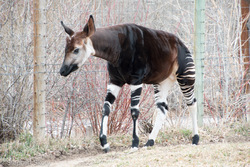
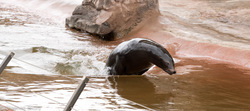
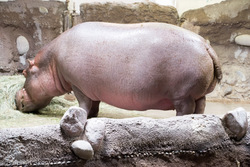
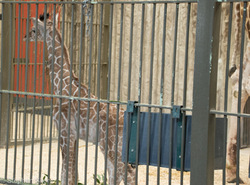
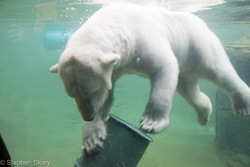
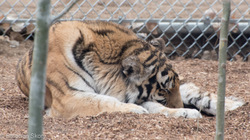
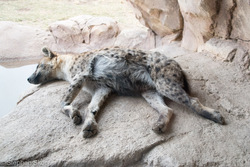
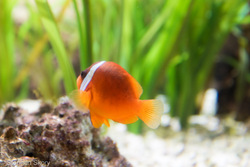
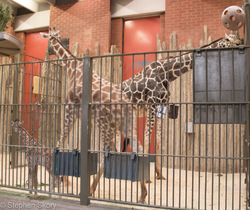
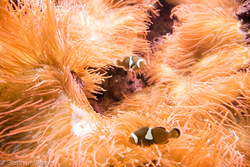
My New Camera
I've been thinking about replacing my 11-year old Konica Minolta Digital SLR for a few months now. The old camera still works okay, but it has issues that started to bother me more and more. The battery door opens on its own, which is annoying. If it hasn't been used for a while (e.g. a few hours), the first photo taken doesn't work and the camera has to be power cycled. There are other signs of wear and tear that all add up to a degraded experience. Sure, it works, but not as well as it used to, and certainly not as well as more modern cameras.
Last week I finally pulled the trigger and purchased a Nikon D5500. An incomplete list of the things this camera does better than the old one:
- The Konica Minolta has 6 megapixels, which is plenty for what I need. The Nikon, with 24 megapixels, probably has more than I need. However, digital sensors have come a long way in a decade. Despite the fact that the Nikon sensor pixels are smaller than the Konica Minolta's (both cameras have APS-C sensors), the Nikon sensor has much better low-light performance than the Konica Minolta.
- The Konica Minolta doesn't have a movie mode, while the Nikon can do 1080P at 60 FPS. I don't often take movies, but it's nice to have that feature there if I want it.
- The Nikon has much faster and better image processing. Of course, this is no Nikon D5 that costs $6,500, but it's plenty fast for my purposes.
- The Nikon has a touch screen that has iPhone-like swipe/pinch gestures, and touch-to-focus. As hilarious as it is to see my daughter try to swipe on my old camera, I like the idea of making photography approachable for her.
- The availability of lenses for Nikon cameras is probably unparalleled. This was a source of consternation for me – let me explain. I have a number of lenses for the Konica Minolta that aren't necessarily top quality, but they work fine. I have coverage from 18-500mm. In 2006 (shortly after I got my Konica Minolta, natch), Sony bought Konica Minolta's camera line, and continue to make cameras to this day based on that product line under their name. Sony still makes cameras that are compatible with Konica Minolta lenses (the A-mount system), but recently they have focused most of their innovation towards their E-mount system. I wasn't sure I wanted to stay with a system that (it looks like to me) Sony isn't focused on. Sony makes a line of very nice mirrorless cameras that only support the E-mount system. I thought long and hard about buying a Sony mirrorless camera (especially the α6500, $1400 for the body), but in the end I decided I couldn't justify spending that much money. With a Nikon, I can get a more reasonably priced body and gain access to a wealth of high-quality lenses.
-
The Nikon can be controlled (in a limited fashion) from a smartphone or tablet over Wifi, which seems pretty useful.
Finally, here's a photo using the new camera from this morning's dog walk. I centered it on the iconic NCAR Mesa Laboratory building. Click for the full 24 megapixel size!
more ...